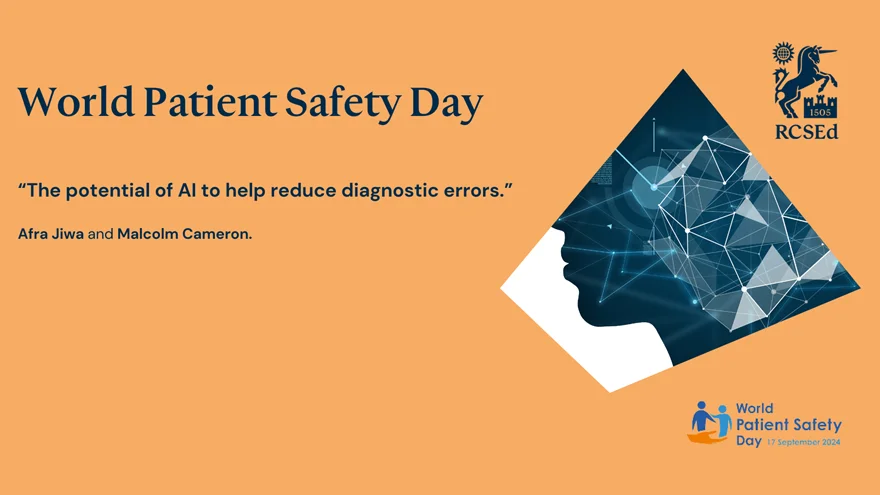
The Patient Safety Group (PSG) of the Royal College of Surgeons of Edinburgh (RCSEd) are delighted to lend our enthusiastic support to the sixth World Patient Safety Day (WPSD). This event, established by the World Health Organisation (WHO) in 2019, takes place on 17 September every year. It helps to raise global awareness amongst all stakeholders about key Patient Safety issues and foster collaboration between patients, health care workers, health care leaders and policy makers to improve patient safety. Each year a new theme is selected to highlight a priority patient safety area for action.
The theme set by the WHO for this year’s WPSD is “Improving diagnosis for patient safety”, recognising the vital importance of correct and timely diagnosis in ensuring patient safety and improving health outcomes.
Diagnostic errors are a significant challenge in the healthcare system, often leading to adverse patient outcomes, increased healthcare costs, and a loss of trust in medical professionals. Studies suggest that diagnostic errors contribute to approximately 10% of patient deaths and up to 17% of adverse events in hospitals. In a field where precision is paramount, even a small margin of error can have catastrophic consequences. Fortunately, advancements in Artificial Intelligence (AI) offer promising solutions to these longstanding issues. AI's ability to process vast amounts of data, identify patterns, and support decision-making processes positions it as a powerful tool in reducing diagnostic errors and improving patient care.
Diagnostic errors can be broadly categorised into the following domains:
- Failure to detect: Missing the presence of disease or condition
- Delayed diagnosis: Identifying the disease or condition, but at a point outstanding ideal diagnostic times
- Misdiagnosis: Failing to detect the correct disease or condition
There are many factors which can lead to diagnostic errors. These can include human factors (cognitive biases, workload and time pressures, communication failures), equipment failures (ageing and outdated technologies) or logistical failures (paper systems, waiting lists, staffing limitations). Certainly, there won’t be a single solution to resolve all these challenges but the rise of increasingly effective AI systems has signalled an opportunity to leapfrog multiple challenges simultaneously and support clinicians to make more accurate and timely diagnoses in a cost-effective way.
The uses of AI in reducing diagnostic errors:
Data Processing and Pattern Recognition
AI relies on large volumes of data which it can then process at relatively high speeds, compared with that of clinicians. By analysing electronic health records, medical images and lab results AI may be able to recognise patterns which are more difficult to perceive by human observers. In an earlier blog on remote diagnostics, we described the need for studies such as the Enhanced monitoring using sensors after surgery (EMUs) which will use physiological data to help detect post-operative complications. One of the secondary outcomes of this study will be to look at time-stamped sensor clinical event data to determine relationships between physiological waveforms and patient deterioration. With the use of AI-predictive algorithms this data can also be used to predict deteriorations based on micro-changes in patient’s waveform data. This would allow for deteriorations to be recognised earlier allowing more time to intervene.
Reducing Cognitive Biases
Human decision-making in healthcare is often influenced by cognitive biases, such as anchoring—where clinicians rely too heavily on initial information—or availability bias, where recent experiences disproportionately shape decision-making. AI can mitigate these biases by offering evidence-based suggestions and highlighting diagnoses that might otherwise be overlooked.
Decision support
AI offers an invaluable ‘re-look’ for clinicians, offering decision support by cross-referencing patient symptoms and test results with historical patient databases. This capability is particularly beneficial in complex cases where multiple conditions might present similar symptoms and relies on consideration of details to differentiate between conditions.
Training and Education
AI has the potential to revolutionize surgical training and education, enhancing patient safety by ensuring that clinicians are optimised to manage everyday conditions. The Creating new models of laparoscopic surgery skills acquisition and assessment (CAMELs) study, which is being run by the University of Edinburgh in association with Wellcome Leap, aims to predict and assess operating room performance based on operative performance ratings derived from box-trainer simulation lab exercises and in-theatre video assessments. By analyzing video data and correlating it with performance metrics, AI can identify specific areas where surgical trainees may need additional practice or instruction, thereby tailoring the training to each individual's needs. In doing so, this work seeks to optimise training pathways for surgeons and ensure that simulated training is optimised to support real-world surgical practice.
While AI holds great promise in reducing diagnostic errors, it is not without challenges and ethical considerations:
- Data Quality and Bias: AI systems are only as good as the data they are trained on. If the training data is biased or incomplete, the AI may produce inaccurate or biased results, potentially leading to new diagnostic errors. This is especially relevant in the context of our diverse patient populations, whom are not always well represented in traditional medical databases2 (most of which are largely composed of data from high-income Caucasian patients). Diversifying training data and ensuring models are available across variably resourced contexts will help alleviate this disparity.
- Transparency and Trust: Clinicians and patients must trust AI-driven diagnostic tools. However, many AI models operate as "black boxes," making it difficult to understand how they arrive at their conclusions. This lack of transparency can be a barrier to adoption and can require a human clinician to take responsibility for AI generated guidance. Questions about accountability for patient safety may come into play as these technologies develop.
- Integration into Clinical Workflows: For AI to be effective, it must be seamlessly integrated into existing clinical workflows. This integration requires significant changes in healthcare infrastructure, training for healthcare professionals and sustainable funding structures.
The potential for AI to significantly reduce diagnostic errors is immense. As AI technologies advance, we can anticipate even greater accuracy, speed, and reliability in diagnostics, potentially leading to a future where diagnostic errors are rare, and patient care is more timely and precise. To realize this potential, the focus must be on improving data quality, ensuring transparency, and building trust among healthcare professionals. With ongoing research, collaboration between AI developers and healthcare providers, and a commitment to addressing ethical concerns, AI is poised to revolutionise diagnostics and substantially reduce the burden of diagnostic errors in healthcare.
Written by Afra Jiwa and Malcolm Cameron
References:
- Committee on Diagnostic Error in Health Care, Board on Health Care Services, Institute of Medicine, The National Academies of Sciences, Engineering, and Medicine. Improving Diagnosis in Health Care. (Balogh EP, Miller BT, Ball JR, eds.). National Academies Press (US); 2015. Accessed August 26, 2024. http://www.ncbi.nlm.nih.gov/books/NBK338596/
- Mittermaier M, Raza MM, Kvedar JC. Bias in AI-based models for medical applications: challenges and mitigation strategies. npj Digit Med. 2023;6(1):1-3. doi:10.1038/s41746-023-00858-z